What is Artificial Intelligence (AI)?
Artificial intelligence (AI) encompasses multi-disciplinary elements to enable machines to perform human-like tasks, with applications in various industries. AI systems range from reactive machines to self-aware systems, while training models include supervised, unsupervised, and reinforcement learning. Key benefits of AI include automation, error reduction, and task efficiency. AI implementation faces growing ethical and regulatory considerations, with future trends focusing on AI's integration with IoT technology, ethical aspects, and human-AI collaboration, among other developments.
ARTIFICIALINTELLIGENCEAICREATIVEAIARTIFICIAL INTELLIGENCEFUTURETRENDSFUTURETECHNOLOGY
Henri Hubert
6/21/20237 min read
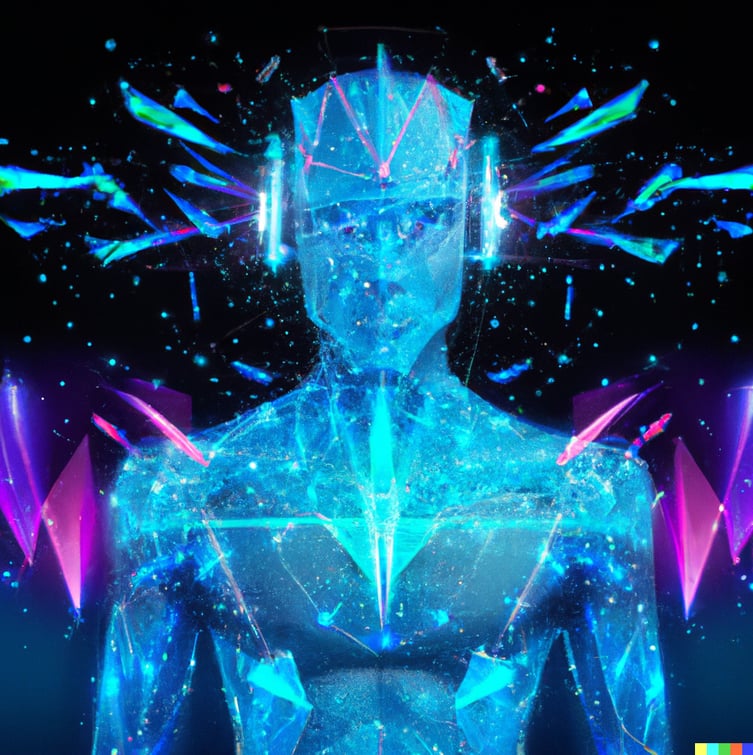
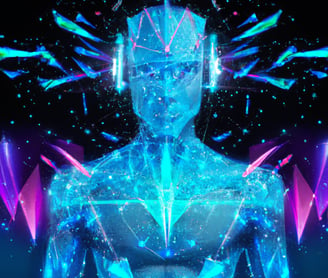
SEO-Meta: Explore the world of Artificial Intelligence (AI): from its multi-disciplinary roots and training models to its widespread applications, benefits, and future trends in various industries.
What is Artificial Intelligence (AI)?
Artificial Intelligence - Unveiling the Science, Applications, and Future Trends
The sphere of artificial intelligence (AI) is ever-evolving, driven by advancements in data analysis, software engineering, neuroscience, and more. In this dynamic milieu, AI emerges as a cornerstone of modern technology, underpinning innovations from autonomous vehicles to speech recognition.
Deciphering Artificial Intelligence (AI)
AI technology is designed to equip machines with capabilities that mirror human intelligence, enabling them to analyze data, understand languages, provide recommendations, and perceive visual information. AI's scope spans multiple disciplines, including computer science, data analytics, hardware and software engineering, linguistics, neuroscience, philosophy, and psychology. In business usage, AI primarily hinges on machine learning (ML) and deep learning, used for applications such as data analytics, predictive forecasting, object classification, natural language processing, and intelligent data retrieval.
Unraveling the Various Types of AI
AI systems can be classified in various ways based on the degree of their sophistication or the functions they perform. There are four widely recognized stages of AI development:
Reactive Machines: AI systems with limited capabilities, responding to different stimuli based on preprogrammed rules. IBM’s Deep Blue, which bested chess champion Garry Kasparov in 1997, is an example of a reactive machine.
Limited Memory: Machines that can improve over time by learning from new data, typically through artificial neural networks. Deep learning, a subset of machine learning, is considered a limited memory AI.
Theory of Mind: This kind of AI, still in the realm of research, aims to simulate the human mind with decision-making capabilities equivalent to that of humans. It can recognize, remember, and respond to emotional cues and social situations like humans.
Self-aware AI: Considered the pinnacle of AI, self-aware machines would possess intellectual and emotional capabilities akin to humans.
AI's capabilities can be categorized as narrow AI, which performs specific tasks based on programming, general AI, capable of understanding and applying knowledge across a broad array of tasks much like a human, and superintelligence, which surpasses human intelligence.
Delving into AI Training Models
Limited-memory AI systems learn and improve by being trained with new data. This training is typically conducted using machine learning, a branch of AI that employs algorithms to analyze data and derive results. Machine learning models can be broadly divided into three categories:
Supervised Learning: Training an algorithm using labeled data to predict outputs from given inputs. For instance, to teach an algorithm to recognize cats, it is trained using images labeled as cats.
Unsupervised Learning: The algorithm identifies patterns in unlabeled data, learning from the data and categorizing it based on attributes. It's suitable for pattern matching and descriptive modeling.
Reinforcement Learning: An AI model learns to perform a specific task through trial and error, with performance enhancing over time through a feedback loop. This method sees positive reinforcement for desired outcomes and negative reinforcement for undesirable ones. An example is training a robotic arm to pick up a ball.
In addition to these, a mixed approach called semi-supervised learning, where some data is labeled, is often used. In this approach, the algorithm must figure out how to organize and structure the data to achieve the desired results.
Understanding Artificial Neural Networks
Artificial Neural Networks (ANNs) constitute a vital part of AI training models. Inspired by the human brain, they consist of interconnected artificial neurons that classify and analyze data. Deep Neural Networks, with more than three layers, form the basis of deep learning, a key subset of AI. Here are some common types of ANNs:
Feedforward Neural Networks (FF): The data flows one way through multiple layers of artificial neurons until an output is achieved. Most modern FF networks use an error-correction algorithm, backpropagation, to improve accuracy.
Recurrent Neural Networks (RNN): RNNs use time-series or sequential data, "remembering" previous layers' inputs, making them suitable for tasks like speech recognition and image captioning.
Long/Short Term Memory (LSTM): An advanced form of RNN, LSTMs remember inputs from several layers ago, making them useful for speech recognition and predictive modeling.
Convolutional Neural Networks (CNN): Predominantly used in image recognition, CNNs use distinct layers to filter different parts of an image before reassembling it.
Generative Adversarial Networks (GAN): Involving two competing networks, GANs improve output accuracy through a game-like setup. One network creates examples that the other network evaluates.
Benefits of AI [1]
AI brings an array of benefits, including:
Automation: AI can automate workflows, monitor and analyze network traffic, or help navigate a factory floor.
Reduced Human Error: AI can minimize errors in data processing and manufacturing through consistent automation and algorithms.
Task Efficiency: AI can take over repetitive tasks, freeing humans to tackle complex problems.
Speed and Accuracy: AI can analyze large volumes of data quickly and accurately.
Availability: Being unhindered by human limitations, AI can operate continuously.
Accelerated R&D: AI's ability to swiftly analyze vast datasets can expedite breakthroughs in research and development.
Demystifying AI Algorithms
Some common AI algorithms include:
Decision Trees: They analyze data through a branching structure to make decisions or predictions. Decision tree algorithms are useful for classification tasks.
Support Vector Machines (SVM): SVM algorithms find an optimal hyperplane that best separates data points into distinct classes. These algorithms are well-suited for complex classification applications.
K-Means Clustering: K-Means is an unsupervised learning algorithm used for clustering tasks by partitioning data points into K clusters based on similarity.
Naïve Bayes: This algorithm is based on the Bayes theorem and predicts class membership probabilities. It's frequently used in text classification tasks, such as spam filtering.
Gradient Boosting and Random Forest: Both are ensemble learning methods that combine multiple weak learning models to create a stronger model. They're particularly effective for regression and classification tasks.
AI's Impact on Key Industries
AI has a profound impact on various industries, transforming traditional methods and introducing novel applications:
Healthcare: AI-driven systems can aid diagnoses, predict patient outcomes, develop personalized treatment plans, and assist in drug discovery [2].
Finance: AI enhances fraud detection, risk assessment, trading algorithms, and customer service through chatbots and natural language processing.
Retail: AI-driven solutions optimize supply chain management, inventory forecasting, and consumer behavior analysis and support personalized marketing campaigns and virtual shopping assistants.
Manufacturing: AI-powered robots streamline production processes, and machine learning models monitor equipment to preemptively identify maintenance needs.
Education: AI enables personalized learning experiences, grading automation, and adaptive content delivery, revolutionizing the traditional education landscape.
AI Regulations and Concerns
The rise of AI has sparked debates about its ethical and regulatory aspects. Recently, the European Union proposed a risk-based approach to AI regulation, classifying AI systems based on their risk to fundamental rights, ranging from unacceptable to high and limited risk [3]. High-risk AI systems are subject to strict requirements, including risk assessment, high-quality datasets to minimize bias, and traceability. Moreover, the regulation establishes the European Artificial Intelligence Board and national competent authorities to oversee compliance. Non-compliance could result in hefty fines. These measures illustrate the growing awareness of AI's potential risks and the need for responsible use.
The Future of AI: Trends and Developments
The future of AI holds great potential, with numerous emerging trends and developments poised to redefine industries and society:
AI and the Internet of Things (IoT): The integration of AI and IoT technologies will enhance automation, improve real-time decision-making, and facilitate predictive maintenance in industries such as manufacturing, transportation, and logistics.
AI Ethics and Regulation: The ethical considerations of AI systems, including bias, transparency, fairness, and responsibility, will increasingly gain prominence and shape AI development and implementation, affecting businesses, governments, and individuals.
Human-AI Collaboration: As AI evolves, humans and AI systems will collaborate more closely to enhance productivity, creativity, and decision-making [4].
AI in Cybersecurity: AI will strengthen cybersecurity defenses through automated threat detection, mitigation, and prevention, safeguarding digital assets and infrastructure [5].
AI Healthcare Revolution: The convergence of AI and healthcare technologies, such as genomics and telemedicine, will enable personalized healthcare delivery and expedite drug discovery, leading to breakthroughs in medical research and patient care [6].
Quantum Computing and AI: The advent of quantum computing promises to significantly accelerate AI's capabilities and enable advancements in areas such as drug discovery, cryptography, and logistics optimization [7]. As quantum computers become more accessible, their impact on AI development will grow exponentially.
AI in Edge Computing: The integration of AI into edge computing devices allows for near real-time decision-making and reduces the latency generally associated with sending data to offsite data centers. This combination will enable a wide range of applications, such as autonomous vehicles, smart cities, and remote patient monitoring [8].
AI in the Entertainment Industry: AI-powered content generation, such as music, art, and virtual reality experiences, is set to redefine and expand the boundaries of artistic expression [9]. Additionally, AI will play an increasing role in personalized entertainment recommendations, adding depth and variety to entertainment consumption experiences.
AI Addressing Climate Change: AI's potential in addressing climate change is gaining attention, with applications such as optimizing energy consumption, monitoring greenhouse gas emissions, and predicting the effects of climate change on ecosystems and infrastructure [10]. These developments could lead to more sustainable practices and resource management across various industries.
AI Democratization: As AI technologies become more accessible and cost-effective, small and medium-sized businesses can harness AI capabilities for decision-making, automation, and innovation [11]. This democratization of AI will likely spur new business models and contribute to the economic and societal impact of this transformative technology.
In conclusion, AI's vast potential across industries, combined with rapid technological advancements, signifies that its impact on society is here to stay. By demystifying the science of AI and addressing its challenges and potential risks, we set the stage for an increasingly connected and automated future.
#ArtificialIntelligence, #MachineLearning, #AIInnovation, #FutureOfAI, #AIEthics, #AIinIndustries, #WhatIsArtificialIntelligence
References:
[1] https://towardsdatascience.com/advantages-and-disadvantages-of-artificial-intelligence-182a5ef6588c
[2] https://bernardmarr.com/27-incredible-examples-of-ai-and-machine-learning-in-practice/
[4] https://hbr.org/2018/07/collaborative-intelligence-humans-and-ai-are-joining-forces
[5] https://www.mandiant.com/resources/reports/m-trends-2023-executive-summary
[6] https://www.ncbi.nlm.nih.gov/pmc/articles/PMC8285156/
[8] https://www.intel.com/content/www/us/en/edge-computing/overview.html
[9] https://builtin.com/artificial-intelligence/generative-ai
[10] https://research.ibm.com/blog/ai-for-climate-change-adaptation